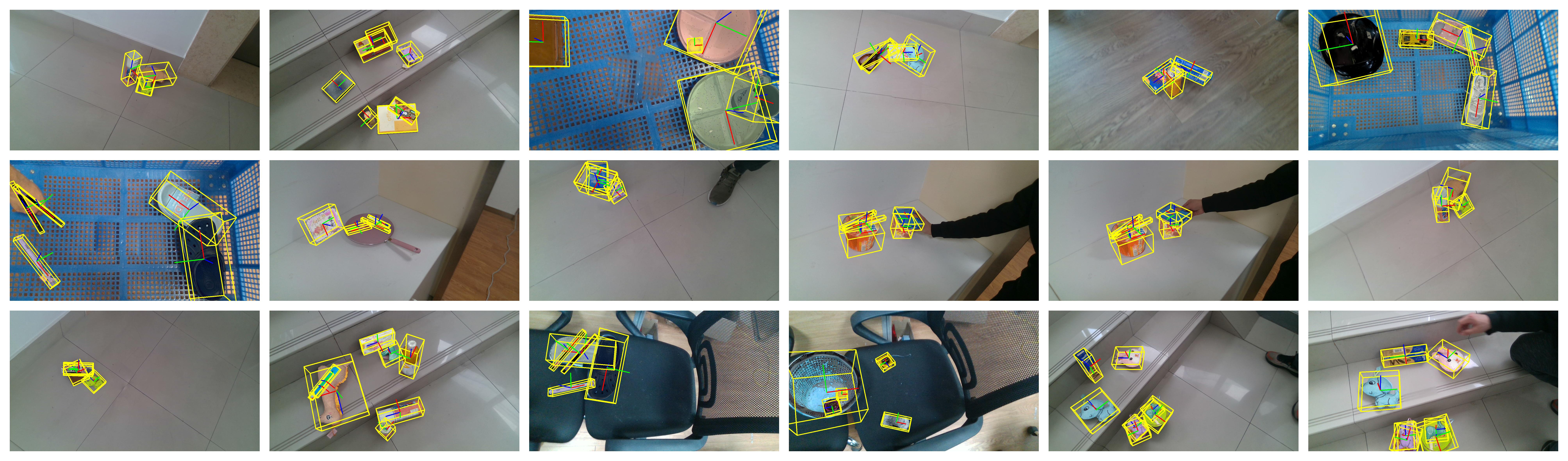
We introduce PACE (Pose Annotations in Cluttered Environments), a large-scale benchmark designed to advance the development and evaluation of pose estimation methods in cluttered scenarios. PACE provides a large-scale real-world benchmark for both instance-level and category-level settings. The benchmark consists of 55K frames with 258K annotations across 300 videos, covering 238 objects from 43 categories and featuring a mix of rigid and articulated items in cluttered scenes. To annotate the real-world data efficiently, we develop an innovative annotation system with a calibrated 3-camera setup. Additionally, we offer PACESim, which contains 100K photo-realistic simulated frames with 2.4M annotations across 931 objects. We test state-of-the-art algorithms in PACE along two tracks: pose estimation, and object pose tracking, revealing the benchmark’s challenges and research opportunities.
Pose Annotation Distrubtion
Object Instance Distrubtion
Object Size Distrubtion
Azimuth and Elevation Distrubtion
Occlusion Distrubtion
Instance-level Pose Estimation
Category-level Pose Estimation
Model-free Pose Tracking
Model-based Pose Tracking
@misc{you2023pace,
title={PACE: Pose Annotations in Cluttered Environments},
author={You, Yang and Xiong, Kai and Yang, Zhening and Huang, Zhengxiang and Zhou, Junwei and Shi, Ruoxi and Fang, Zhou and Harley, Adam W. and Guibas, Leonidas and Lu, Cewu},
booktitle={European Conference on Computer Vision},
year={2024},
organization={Springer}
}